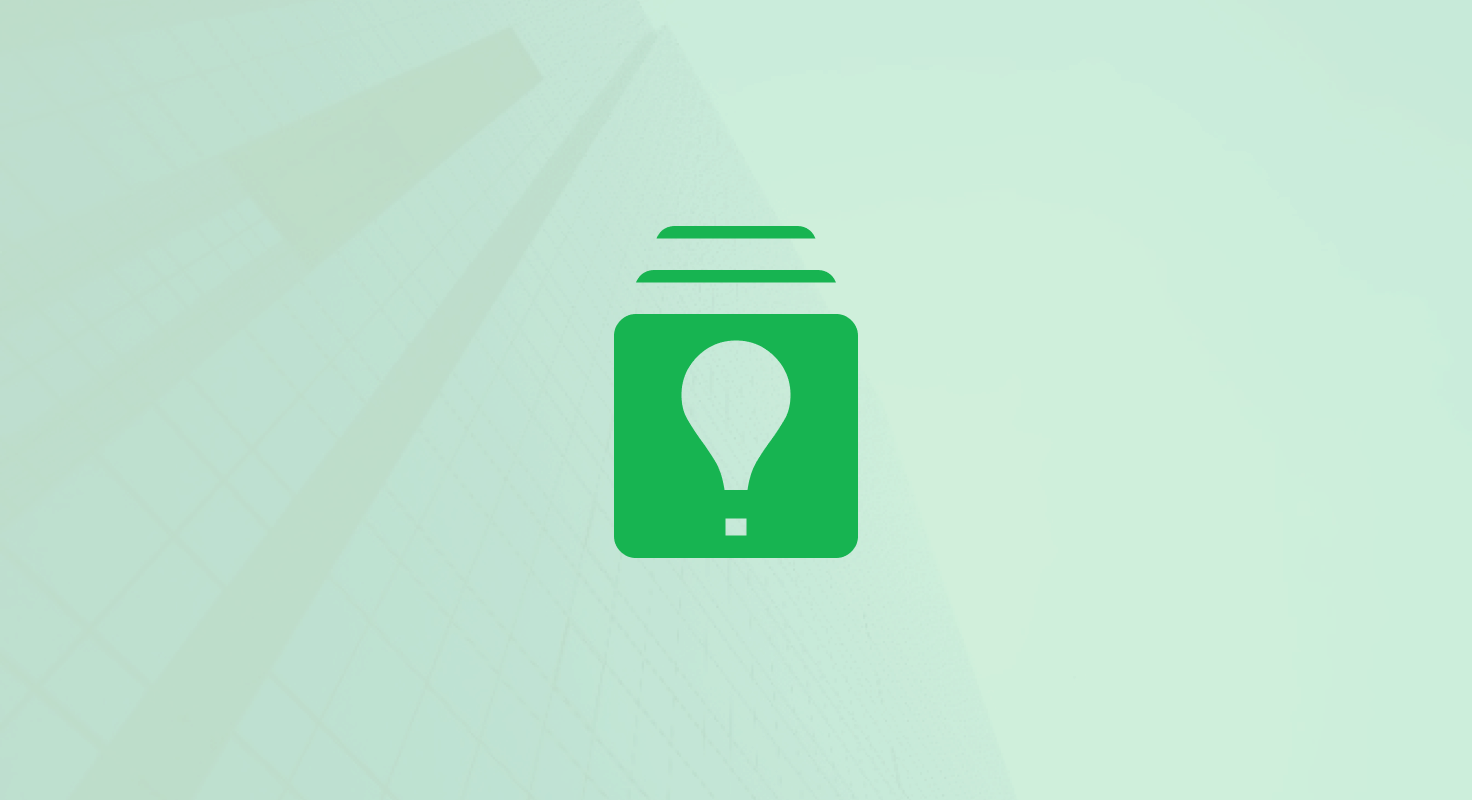
When customers churn (leave), it can have a significant impact on the success and growth of a company.
One way to mitigate the risk of high churn rates is to improve your ability to predict it. Accurately predicting customer churn is essential as it allows businesses to identify potential risks, implement targeted retention strategies, and foster long-term customer loyalty.
Implementing HubSpot's onboarding process can help configure essential tools for accurate churn prediction and data analysis right from the start.
Effective Customer Churn Analysis
A thorough customer churn analysis enables businesses to identify patterns and data, understand the reasons behind customer loss, and develop strategies to reduce customer churn.
Identifying Key Indicators of Churn
- Decreased usage or engagement: Monitor changes in user activity levels, such as a decline in logins, page views, or time spent on the platform. Identify thresholds that signal potential churn risks and monitor customer behavior closely when these thresholds are crossed.
- Negative customer feedback: Pay attention to complaints, poor reviews, or dissatisfaction expressed through support channels, social media, or online forums. Integrating sentiment analysis tools with HubSpot enables real-time tracking of customer feedback, making it easier to address churn risks effectively.
- Payment or subscription issues: Be vigilant about delayed payments, declined credit cards, or downgrades in subscription plans. Consider implementing automated alerts for payment issues to identify potential churn risks early on.
- Customer service interactions: Analyze the frequency and nature of customer support interactions, as increased support requests or unresolved issues may indicate a higher likelihood of churn.
Analyzing Customer Behavior Patterns
- Lifecycle stages: Segment customers by their lifecycle stages (e.g., awareness, consideration, purchase, retention, advocacy) and analyze churn rates for each stage to pinpoint critical points where intervention is needed.
- Demographic and psychographic factors: Investigate if specific customer segments (age, gender, location, interests) are more prone to churn and adjust your retention strategies accordingly.
- Conversion rates: Monitor conversion rates across various touchpoints and stages of the customer journey to identify potential bottlenecks or drop-offs that may lead to churn.
Segmenting Customers for Targeted Customer Churn Analysis
- Customer value: Segment customers based on their lifetime value and identify high-value customers who contribute significantly to your revenue. Prioritize retention efforts for these customers to minimize the impact of potential churn.
- Engagement levels: Group customers by their engagement levels (low, medium, high) to identify at-risk customers who require more personalized attention or re-engagement campaigns.
- Product or service usage: Segment customers based on their product usage patterns, such as power users, occasional users, or dormant users. Tailor retention strategies to cater to the unique needs and preferences of each user group.
- Acquisition channels: Analyze customer churn rates by acquisition channel to identify those that yield higher-quality customers and optimize your marketing efforts accordingly.
Building a Customer Churn Prediction Model
An accurate customer churn prediction model enables businesses to forecast customer churn likelihood and take proactive measures to retain existing customers.
Main Components of a Churn Prediction Model
- Identifying relevant data: Select features or variables that can impact customer churn, such as demographics (age, location), engagement levels (login frequency), or billing information (subscription plan). The primary goal is to predict whether a customer will churn (1) or not (0).
- Choosing a methos to predict churn: Pick a suitable algorithm, like logistic regression, decision trees, random forests, or neural networks. Consider your data and business objectives when choosing the best approach.
- Preparing your data: Clean, transform, and preprocess your data to boost model performance. This may involve handling missing values, encoding categorical variables, or scaling continuous variables. Proper data preparation significantly enhances model performance and reduces bias.
- Feature selection and enhancement: Select relevant features and create new ones to improve prediction accuracy. Techniques like correlation analysis or recursive feature elimination help pinpoint influential variables, while feature engineering methods capture complex relationships and interactions between variables. HubSpot technical consulting can help refine data models and ensure accurate feature selection, improving the performance of churn prediction models.
Comparing Popular Prediction Algorithms
- Logistic regression: Works well for simple and complex datasets but might struggle with non-linear relationships. Provides interpretable results and can be regularized to avoid overfitting.
- Decision trees: Easy-to-interpret models that split data into subsets based on feature values. Overfitting can be an issue if the model is not simplified or regulated.
- Random forests: Combine multiple decision trees to reduce overfitting and improve generalization. Highly adaptable to different data types but might require optimization techniques for large datasets.
- Neural networks: Deep learning approach that excels at identifying complex patterns and relationships through layered structures. Requires substantial data and computational resources, with regularization techniques to prevent overfitting.
Integrating Predictive Analytics into Your Business Strategy
Proactive Customer Retention Strategies
- Personalized offers and incentives: Leverage customer data to create targeted promotions, discounts, or loyalty programs tailored to individual preferences and usage patterns.
For instance, use collaborative filtering or content-based filtering techniques to offer personalized product recommendations based on past purchases. Additionally, implement personalized pricing strategies or provide special offers for customers who haven't made a purchase in a while to encourage engagement and reduce churn. - Enhanced customer support: Use predictive analytics to identify customers experiencing issues or expressing dissatisfaction and prioritize support efforts accordingly. Offer proactive support, such as automated issue resolution or dedicated support channels for high-risk customers, to address problems before they lead to churn. Implement real-time customer support analytics to monitor support performance, track common issues, and continuously improve support processes to enhance customer satisfaction and loyalty.
Resource Allocation
- Prioritizing high-value customers: Focus retention efforts on high-value customers, such as those with high lifetime value or significant growth potential, by offering exclusive benefits, personalized service, or dedicated account management.
Implement customer lifetime value models to identify high-value customers and allocate marketing, sales, and support resources accordingly. Additionally, use predictive analytics to identify customers with high upsell or cross-sell potential and tailor retention strategies to maximize revenue and growth. - Reducing investment in low-potential customers: Use predictive analytics to identify customers with low engagement or retention potential and reduce marketing or support investments in these segments. Segment customers based on engagement levels, retention probability, or profitability and allocate resources more effectively by focusing on customers with higher growth potential. Implement customer segmentation models and RFM (recency, frequency, monetary value) analysis to identify low-value or dormant customers and optimize resource allocation across different customer segments.
Adapting to Market Trends and Changes
- Monitoring industry shifts: Keep track of market trends, competitor strategies, and emerging technologies that may impact customer churn rates or preferences. Use this information to refine your churn prediction model and adapt retention strategies accordingly. Conduct market research and competitive analysis to stay informed about industry developments and potential threats. Implement customer feedback loops and voice-of-the-customer programs to gather insights on customer needs and preferences, helping you refine your churn prediction model and adapt retention strategies to align with market changes.
- Continuous model refinement: Regularly update and retrain your churn prediction model to incorporate new data points, features, or algorithms that better reflect current market conditions and customer behavior. This helps maintain model accuracy and relevance over time. Implement model monitoring and evaluation processes to track model performance and identify opportunities for improvement. Experiment with new machine learning algorithms, feature engineering techniques, or data sources to enhance model accuracy and adaptability to changing market conditions.
Want to learn the blueprint to optimizing revenue?
Download our whitepaper on the 4 levers of revenue growth