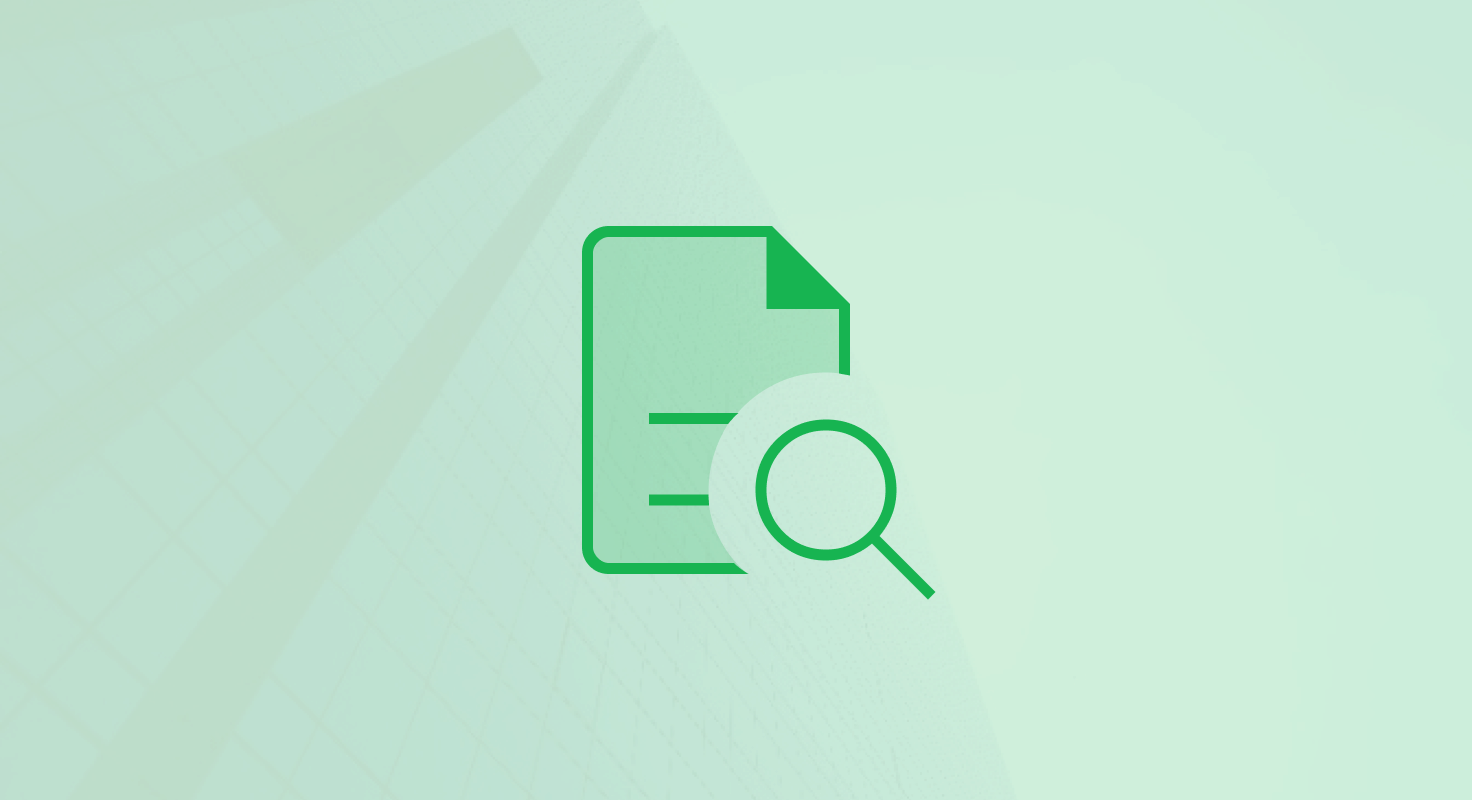
Accurate SaaS revenue forecasting is essential for success. As SaaS companies rely heavily on recurring subscription revenue, accurate forecasting allows them to make informed decisions and plan for the future. However, revenue forecasting isn’t easy and presents significant challenges.
Let’s take a look at the strategies that can help SaaS companies achieve more accurate revenue forecasts and the tools and technologies available to support this process.
Understanding SaaS Revenue Forecasting
Before getting into the strategies, it's important to have a solid understanding of SaaS revenue forecasting. In simple terms, it involves predicting the amount of money a SaaS company will earn from its customers over a specific time period. This forecast is typically based on historical data, trends, market conditions, and other relevant factors.
To create an accurate revenue forecast, companies need to consider multiple variables, such as customer acquisition rate, churn rate, expansion revenue, and pricing changes. By analyzing these factors, businesses can estimate how their revenue will evolve over time and make data-driven decisions.
Challenges in SaaS Revenue Forecasting
Data Quality and Availability
One major challenge in SaaS revenue forecasting is ensuring the quality and availability of data. Accurate forecasting requires reliable historical data, but there can be issues with data quality, such as:
- Incomplete data: Missing data points can skew forecasting models and lead to inaccurate predictions.
- Inconsistent data: Differences in data collection methods, data sources, or data entry errors can result in inconsistent data, making it difficult to analyze and forecast accurately.
- Outdated data: SaaS companies operate in a dynamic environment, and using outdated data can lead to inaccurate forecasts.
To address these challenges, companies should:
- Establish data governance policies: This ensures that data is collected, stored, and managed consistently across the organization.
- Implement data quality checks: Regularly auditing data for completeness, consistency, and accuracy can help identify and address data quality issues.
- Leverage data integration tools: Using data integration tools can help consolidate data from different sources into a unified dataset for analysis.
- Automate data collection processes: Automating data collection can reduce human errors and ensure that data is up-to-date.
Evolving Market Conditions
SaaS companies operate in a dynamic market, and changing conditions can impact revenue forecasting. Key factors include:
- Customer behavior: Changes in customer preferences, buying patterns, and usage habits can impact revenue.
- Competitive landscape: New entrants, product innovations, and pricing changes by competitors can affect market dynamics.
- Economic conditions: Fluctuations in economic conditions, such as inflation, interest rates, and consumer confidence, can impact demand for SaaS products.
To adapt to evolving market conditions, companies should:
- Conduct regular market research: This can help identify shifts in customer behavior, competitor activities, and market trends.
- Use scenario planning: This involves developing multiple forecasting models based on different assumptions and scenarios to assess potential impacts on revenue.
- Monitor economic indicators: Tracking economic indicators can help companies anticipate and adapt to economic fluctuations.
- Develop flexible forecasting models: Flexible models that can be adjusted based on changing market conditions are essential for maintaining accuracy in revenue forecasts.
Customer Churn and Expansion Revenue
One of the key challenges in SaaS revenue forecasting is understanding and managing customer churn and expansion revenue. Customer churn refers to the rate at which customers cancel or downgrade their subscriptions, while expansion revenue comes from upselling, cross-selling, and contract renewals.
Here are some factors to consider when addressing these challenges:
- Accurately estimating churn rates: Understanding the underlying drivers of churn, such as product satisfaction, customer support quality, and pricing competitiveness, is essential for developing effective retention strategies.
- Identifying opportunities for expansion revenue: Leveraging customer data and analytics can help companies identify opportunities for upselling and cross-selling, as well as optimizing pricing strategies based on factors like customer willingness to pay and the value of different product features.
- Conducting regular customer satisfaction surveys: This can help identify areas for improvement and potential drivers of churn.
- Implementing customer feedback loops: This can help companies address customer concerns and improve their products and services, which can lead to increased customer satisfaction and decreased churn.
- Developing customer retention strategies: This can include personalized marketing, customer success programs, and loyalty incentives. By focusing on customer retention, companies can not only increase expansion revenue but also improve overall customer lifetime value.
Strategies for Accurate SaaS Revenue Forecasting
Historical Data Analysis
One effective strategy is to analyze historical data to identify trends and patterns. By examining past revenue performance, companies can gain insights into the behavior of different customer segments, understand the impact of seasonality, and detect any anomalies or outliers.
However, it's not enough to simply look at the numbers. SaaS companies need to dig deeper and understand the underlying factors that drove those revenue trends. Was it a result of a successful marketing campaign? Did a new feature release contribute to increased customer acquisition? By understanding the context behind the numbers, companies can make more accurate forecasts.
It's also essential to leverage advanced analytics tools to conduct this analysis efficiently. These tools can help identify hidden patterns and provide visualizations that make it easier to interpret the data and derive actionable insights.
Collaboration between Sales and Finance Teams
Close collaboration between the sales and finance teams is crucial for accurate forecasting. Sales teams have valuable insights into customer behavior and market trends, which can help refine revenue projections. By aligning the efforts of these two departments and fostering transparent communication, companies can enhance forecasting accuracy.
By regularly sharing information and feedback, the sales team can provide valuable input to the finance team, enabling them to make more accurate revenue forecasts. For example, the sales team can provide insights into customer preferences, buying patterns, and upcoming deals, which can be incorporated into the forecasting models. Similarly, the finance team can share their understanding of market conditions, pricing strategies, and revenue goals, allowing the sales team to align their efforts accordingly
Cohort Analysis
Cohort analysis is another valuable strategy for SaaS revenue forecasting. By grouping customers based on common characteristics, such as the month they signed up or their geographical location, companies can understand how different cohorts behave over time.
However, it's important to go beyond the basic cohort analysis and consider additional variables that may impact revenue. For example, analyzing cohorts based on customer size or industry can provide valuable insights into revenue growth potential within specific market segments.
This analysis can reveal valuable information about customer retention rates, lifetime value, and revenue growth. By tracking the performance of cohorts and applying these insights to future projections, companies can achieve more accurate revenue forecasts.
Scenario Modeling
By creating multiple scenarios based on different assumptions, companies can evaluate the potential impact of various factors on their revenue projections.
For example, by simulating the effects of changes in customer acquisition rates or pricing strategies, companies can assess best-case, worst-case, and most-likely scenarios. This approach allows for more informed decision-making and enables companies to develop contingency plans.
However, it's important to strike a balance between being conservative and realistic in scenario modeling. While it's crucial to consider potential risks and challenges, overly pessimistic scenarios may hinder growth opportunities and innovation.
Constant Monitoring and Iterative Forecasting
Revenue forecasting is not a one-time activity. To achieve accuracy, it requires constant monitoring and iterative forecasting. Companies should regularly review and update their forecasts based on new data and market conditions. By staying agile and responsive, businesses can adapt their strategies and make necessary adjustments to achieve accurate revenue forecasts.
By continuously monitoring KPIs such as customer acquisition, churn rate, and average revenue per user, companies can identify any deviations from their forecasts and take corrective actions.
Tools and Technologies for SaaS Revenue Forecasting
To support these strategies, various tools and technologies are available to facilitate accurate SaaS revenue forecasting.
Business Intelligence (BI) Platforms
BI platforms, such as Tableau and Power BI, provide robust analytics capabilities that help companies visualize and analyze revenue data. These platforms enable companies to identify trends, perform scenario analysis, and generate accurate forecasts. With interactive dashboards and data visualization tools, businesses can easily spot patterns and anomalies in their revenue data, allowing for more informed decision-making. BI platforms also allow for easy data exploration and ad-hoc analysis, empowering users to dive deeper into their revenue data and uncover hidden insights.
CRM Systems
CRM systems, like HubSpot, offer valuable insights into customer behavior and can be integrated with other forecasting tools. These systems provide a centralized repository for customer data, making it easier to track and analyze customer interactions and revenue streams.
With a CRM system, SaaS companies can track key metrics such as customer acquisition costs, customer lifetime value, and churn rates. By analyzing this data, companies can identify patterns and trends that impact revenue forecasting, allowing them to make more informed decisions.
Data Integration Tools
Data integration tools, like Zapier, can help companies consolidate data from various sources, ensuring the accuracy and completeness of their forecasting models. These tools streamline data extraction, transformation, and loading processes, allowing for more reliable forecasts. By automating data integration, businesses can eliminate manual data entry and reduce the risk of data inconsistencies. These tools also offer data validation and cleansing capabilities, ensuring that the data used for forecasting is accurate and reliable.
Summing Up SaaS Revenue Forecasting
SaaS companies must prioritize accurate revenue forecasting to stay competitive, drive growth, and achieve long-term success. By leveraging advanced technologies, embracing a data-driven approach, and adopting best practices in forecasting, companies can navigate the challenges which inevitably come up.
Want to learn the blueprint to optimizing revenue?
Download our whitepaper on the 4 levers of revenue growth